ABSTRACT
Design and optimization are pivotal in a pharmaceutical company’s formulation and manufacturing process. Various approaches and methodologies are employed in formulation design. A quantitative adjustment to a component, ingredient, or process variable is made while maintaining control over other independent components to monitor their influence on quality attributes. However, investigating these components together poses challenges, potentially leading to misinterpretation of experimental results. To resolve this, simultaneous design and development process modification can be achieved using the design of experiment methodologies, such as Central Composite Design (CCD). CCD effectively evaluates the interaction effects of numerous elements on product quality and outcomes. It has been successfully applied across various studies to develop and enhance formulations. This review provides insights into CCD, including its varieties, a comparison with other experimental designs, applications in pharmaceutical formulation, experimental design data analysis, optimization techniques, benefits and advantages, case studies, integration with Quality by Design (QbD) and regulatory considerations.
INTRODUCTION
CCD as A Statistical Optimization Technique
Design and optimization are the most significant steps in creating a recommended system or formulation. In formulation design, many different approaches and methodologies are used.1 Quantitative analysis of one component or process variable while controlling others’ levels to monitor quality. On the other hand, the approach calls for a significant amount of investigation and it is difficult to investigate those components when they function together and the results of the experiment might be misinterpreted.2 CCD has been successfully used in various studies to develop and enhance formulations. This is because the data generated by CCD reveal accurate and reliable predictions. Statistical and experimental research methods are often used in the Design of Experiments (DOE).3 The main goal of the CCD technique is to effectively analyze the impacts of categorical and continuous factors on a response variable while reducing the number of required experimental runs. Integration of factorial, center and axial points is performed to achieve this goal.4 The data obtained by CCD provides accurate predictions and this method had appreciable results in several studies of formulating and improving formulations.5 When designing a tablet formulation, CCD was used in conjunction with two independent parameters (the ratio of excipients to drug concentration) at two different levels (low and high) to build the formulations. In CCD, trials are categorized into factorial, axial and central points based on the parameters for design qualities the experiment demands. The angle of repose, disintegration time and drug release from tablets are crucial variables in CCD optimization. Choosing the right experimental design can be challenging. The CCD is a significant option in optimizing ongoing batches to identify the best potential product.6 CCD surface models enhance experimental data analysis, offering insight into complex variable relationships for optimization. A collection of “star points” and “center points” are included in the CCD model, which is the Central Composite Design by Box and Wilson.7 The review explores Central Composite Design (CCD), covering its types, comparisons, applications in pharmaceuticals, optimization techniques, benefits, case studies and regulatory aspects. After exclusion, 67 documents were included in the review (Figure 1).
Historical Context of CCD
Both experimental design and response surface technique are the foundations upon which CCD was built. The CCD continues to be important in various domains due to its attempts to improve the efficiency of experiments, particularly in research with several different variables and complex relationships.
Experimental Design
Sir Ronald A. Fisher, a British statistician, is widely recognized for his groundbreaking contributions to the Design of Experiments (DOE) field in the early 20th century. Fisher’s work is considered foundational to DOE, providing a framework for systematically studying the effects of multiple factors in an experiment.8 One of his key innovations was introducing these factorial designs, which revolutionized the field by allowing more efficient and comprehensive experimentation.
Response Surface Methodology (RSM)
RSM was formulated in the mid-20th century as a suite of statistical and mathematical tools employed to model and refine responses influenced by multiple variables. George E.P. Box and K.B. Wilson played pivotal roles in advancing RSM during the 1950s and 1960s. Daniel aimed to improve the effectiveness of experiments by strategically placing experimental points within the design space encompassing a mix of central, factorial and axial points.9
Pharmaceutical Formulation Development
CCD plays a significant role in improving medication formulations within the pharmaceutical industry.10 Central Composite Design (CCD) optimizes drug formulations by exploring excipient ratios and processing conditions, reducing trials and enhancing efficacy, safety and bioavailability in pharmaceutical research It contributes to the development of high-quality and cost-effective drug products.11
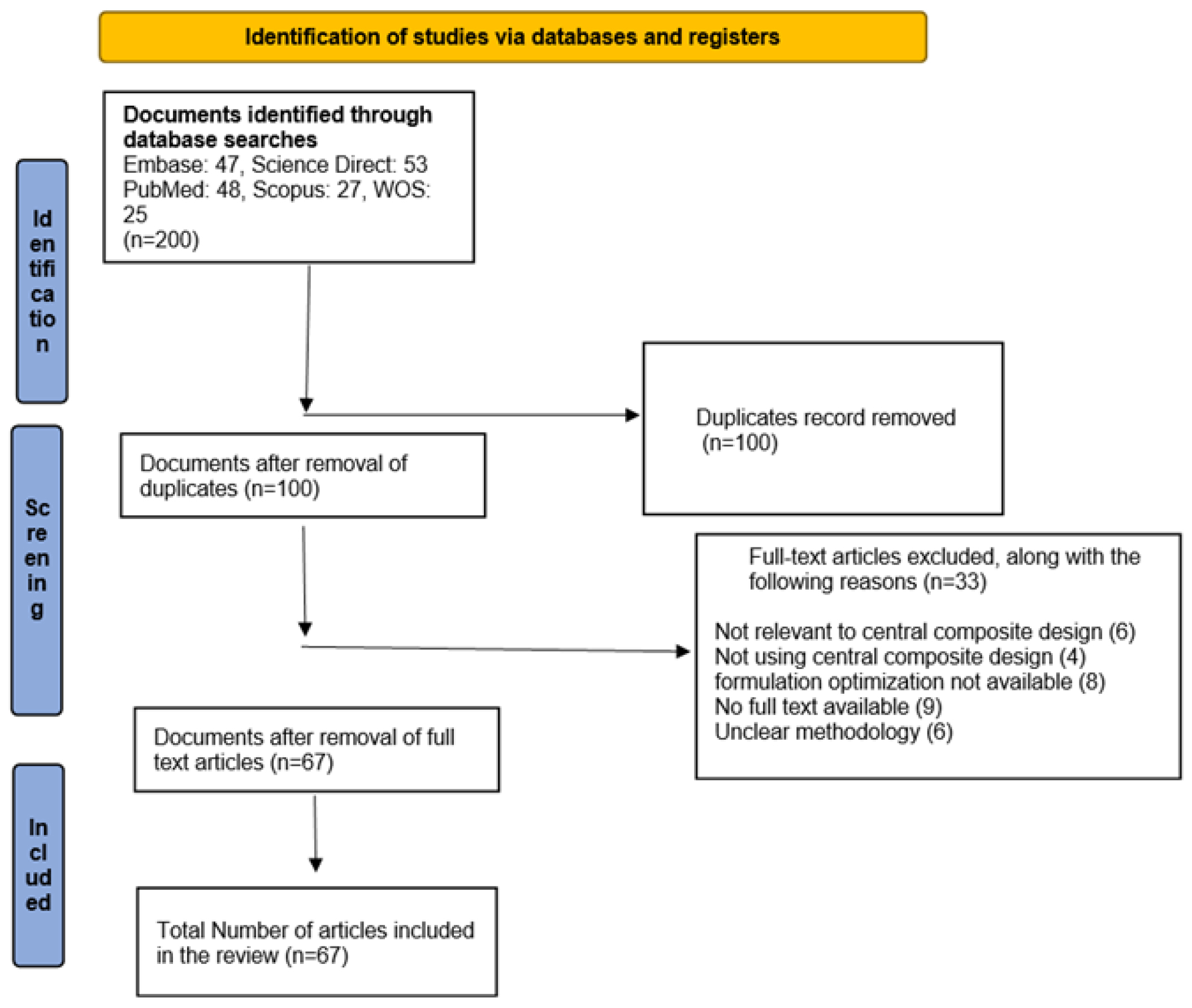
Figure 1:
Prisma chart.
Principles and Components of CCD
CCD of Linear and Quadratic Effects
The mathematical model of CCD can integrate both linear and quadratic influencing factors, resulting in a response surface representation that better fits real experimental data. During the analysis, the coefficients of these variables are calculated, aiding researchers in understanding the relationship between factors and the response. This comprehensive modeling of linear and quadratic effects enhances the ability to optimize and predict responses within the experimental design space.12 The linear and quadratic effects describe the different components in the model, providing a more holistic understanding of various conditions that influence the response.
Linear Effects
Linear effects indicate the direct relationships between individual factors and the response variable, showing how the response changes as each factor varies. Each factor has a linear term, such as ‘bX,’ where ‘b’ is the coefficient representing the change in the response for a unit change in ‘X.’
Quadratic Effects
‘Quadratic effects’ refers to curvature or non-linear behavior in the relationship between causes and responses. This model captures the curvature of the response surface through specific terms. For instance, the quadratic effect for a factor ‘X’ can be expressed as ‘cX2,’ with ‘c’ representing the coefficient that captures the curvature effect.
Parts of CCD
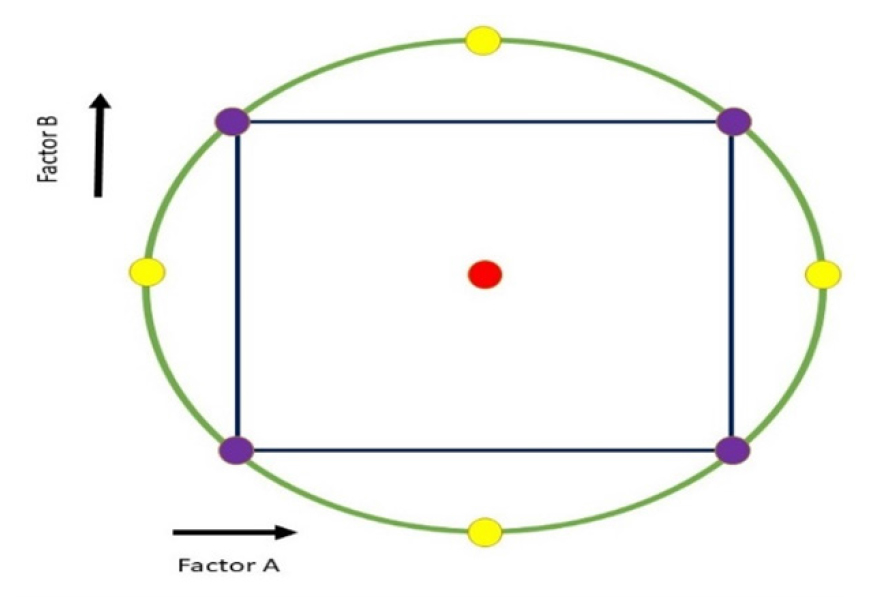
Figure 2:
Circumscribed design of Central Composite Design.
Factorial points allow for estimating main effects, linear interaction and quadratic effects by covering combinations at two levels (#1, þ1). They are essential in the process of optimization. Center points, denoted by the level “0,” typically range from three to five and are crucial for generating quadratic model terms and analyzing quadratic interaction effects. The value of “α” ensures design symmetry and indicates its rotatability. CCDs can include two to fifty factors, each with five levels (-α, þα, #1, 0 and þ1). The number of experiments can be determined using the following equation:13
K is the number of factors, 2 k is the number of factorial points, 2K is the number of axial/star points and C0 is the number of center points.
CCD Varieties
CCDs can be classified into two main types depending on how axial/star points are arranged.
Composite design with a Rotatable Center support (RCCD)
In the RCCD design, the axial points are positioned at a distance (α) from the center of the design and are not aligned with the plane of the factorial points. Unlike the faces of the factorial cube, these points may extend beyond those faces.14 A key advantage of this design is its ability to be rotated, ensuring that the variance prediction remains consistent at any location equidistant from the center. This characteristic is essential for ensuring a robust and reliable analysis of experimental data.
Circumscribed Design (CCCD)
The star points within this model establish new boundaries for all factors’ lowest and highest settings. This five-level representation is essential for circular, spherical, or hyper-spherical symmetry designs (Figure 2). To create such a design, a star point can be added to an existing factorial or factor design and has demonstrated that the CCCD pattern is rotatable.15
Inscribed design (CCI)
The inscribed design (CCI) context utilizes limited factor settings as star points, effectively creating a factorial design within predetermined boundaries. Essentially, the CCI design is a variant of the CCC design, achieved by dividing the CCC design by α to create the CCI model (Figure 3). Consequently, it has been noted that CCC and CCI are examples of rotating models.16
Central composite design with the face as the focal point
In the Face-centered Central Composite Design connecting the axial points to the factorial points’ plane involves situating these axial points on the faces of the factorial cube at a specific distance (α) from the design’s center noted that in this design, the axial points coincide with the midpoint of each face of the factorial space, aligning the levels #1/þ1 for the factorial points with the axial points and placing both sets of points on the same plane.17 FCCD provides insights into linear and interaction effects of components, efficiently estimating main effects and interactions, strategically positioning design points for effective factor space investigation Figure 4.
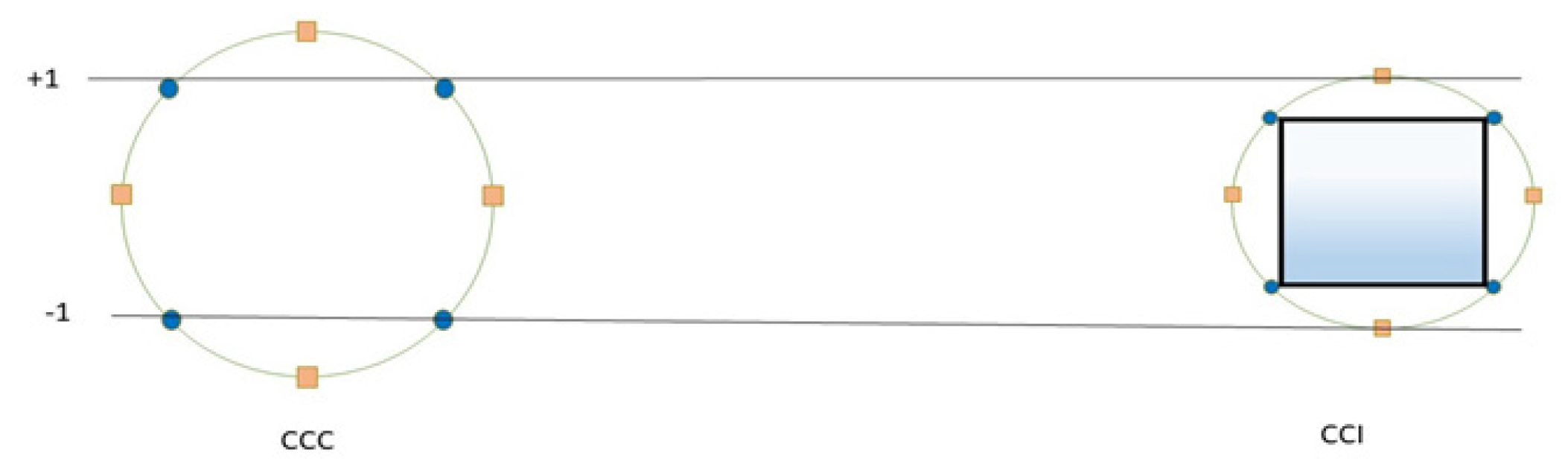
Figure 3:
Inscribed design of Central Composite Design.
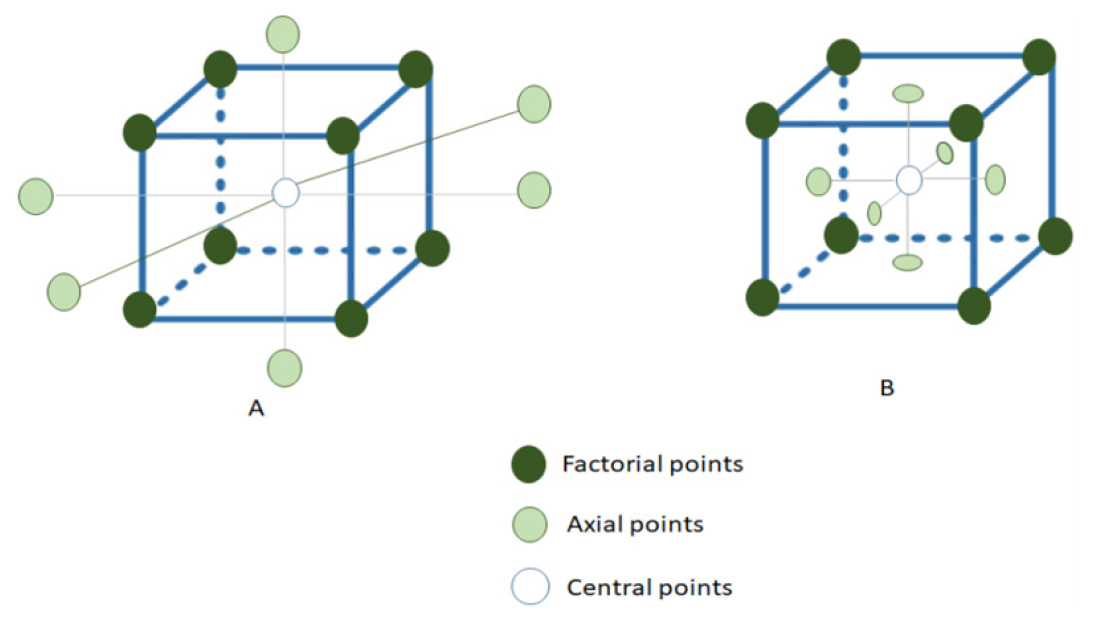
Figure 4:
Face-centered Central Composite Design of central composite design. (a): Rotatable Central Composite Design (RCCD), (b): Face-Centered Central Composite Design (FCCD).
Alpha value
In the CCD, a star point’s distance from the center equals α, where α is less than eight. The actual value of Alpha (α) is established according to the exact design requirements. Adding more than two or multiple star points is possible, given the various parameters included in the CCD model.18
Comparison with Other Experimental Designs
The CCD model extends two-level factors commonly employed in response surface modeling and optimization. In recent years, significant scientific inquiry has been directed toward this approach in pharmaceutical research.
Applications in Pharmaceutical Formulation
Recent Studies and Applications of CCD in Pharmaceutical Formulation Development
Researchers in pharmaceutical sciences are continually exploring and adapting experimental designs, including CCDs, to tackle the complexities of drug development and manufacturing.19 Staying abreast of recent literature and publications is needed for this field’s latest applications and advancements. Drug Formulation Optimization by CCDs is extensively used to optimize drug formulations, considering factors like excipient composition, drug concentration and processing parameters. They help identify the optimal conditions for achieving desired drug release profiles and enhancing bioavailability. Quality by Design (QbD) in Process Development by pharmaceutical companies increasingly embraces CCDs and is vital for a crucial role in process development.20 Biopharmaceuticals and protein Formulations development employs CCDs in developing biopharmaceuticals and protein formulations to optimize conditions for protein stability, folding and aggregation. This optimization is critical for ensuring the efficacy and safety of biologic drugs.21 Nanoparticle formulation and drug delivery systems use CCDs to optimize the formulation of nanoparticles and various drug delivery systems, including factors like particle size, surface charge and drug loading.22 This optimization aims to improve drug delivery and therapeutic outcomes. Analytical method development in the pharmaceutical industry must be robust and reliable. CCDs are occasionally employed in method development to optimize conditions for analytical techniques, ensuring accurate and precise analysis of pharmaceutical products. Solubility enhancement studies using CCDs explore and optimize strategies for enhancing the solubility of poorly water-soluble drugs.23 This optimization is crucial for improving the bioavailability of such drugs, especially in early drug development stages. CCDs are applied in pharmaceutical manufacturing to optimize various unit operations, such as tablet compression, granulation and coating processes.24 This optimization contributes to efficient and cost-effective manufacturing processes.
CCD for Optimize Drug Solubility, Bioavailability, Stability and Other Critical Formulation
CCDs have emerged as a valuable tool in pharmaceutical research, particularly for optimizing drug formulations to improve solubility and stability. CCDs comprehensively provides understanding of non-linear relationships by systematically exploring factors, such as excipient composition, drug concentration and processing conditions, including low, high, axial and center points. This versatile experimental design is essential in numerous applications, including lipid-based nanoparticle formulation, oral drug development, protein stability studies and controlled-release drug delivery systems.25 In nanoparticle formulation, CCDs help determine optimal conditions for size, encapsulation efficiency and release kinetics.26 CCDs optimize excipient composition and pH for oral drugs to enhance solubility and absorption. CCDs contribute to protein stability by varying buffer composition and temperature.27
Examples of Specific Drug Formulations or Delivery Systems Optimized Using CCD
The CCD method is extensively used in pharmaceutical formulation development to optimize drug formulations and delivery systems. This approach has been applied in various areas, such as controlled-release tablets, liposomal drug delivery systems and Nano particulate drug delivery systems, etc.,28 In controlled-release tablets, CCD helps to adjust factors like polymer types, concentrations and processing conditions for sustained and predictable drug release over time and balancing therapeutic efficacy with patient compliance. Similarly, in liposomal drug delivery systems, CCD is crucial for optimizing lipid composition, drug-to-lipid ratio and preparation methods to enhance drug encapsulation efficiency and target specific tissues.29 Nano particulate drug delivery systems also benefit from CCD, which gets optimized for dependable factors like polymer type, drug loading and particle size to achieve optimal drug release kinetics and bioavailability (Table 1). CCD is also applied in the optimization of transdermal patches, orally disintegrating tablets, inhaled drug formulations, emulsion-based formulations, polymeric microspheres for injectable drug delivery, ophthalmic drug delivery systems, gastro retentive drug delivery systems, hydrogels for topical delivery, suppositories and inserts and buccal drug delivery systems.30
Drug deliveries | CCD Application studies | Variables (Independent) | Responses |
---|---|---|---|
Controlled-Release Tablets. | Formulation and Optimization of controlled-release mucoadhesive Tablets of Atenolol Using Response Surface Methodology.31 | Carbopol 934P (cp) and Sodium carboxymethylcellulose. | CCD optimized Atenolol tablets for controlled release. |
Liposomal Drug Delivery Systems. | Optimization of the process variables of tilianin-loaded composite phospholipid liposomes based on response surface-central composite design and pharmacokinetic study.32 | Phospholipids, Cholesterol and Weight ratio of phospholipid to drug. | Response surface-CCD optimizes drug delivery by altering lipids. |
Nanoparticulate Drug Delivery Systems. | Development and optimization of solid lipid nanoparticles of amikacin by central composite design.33 | The ratio of the drug to lipid, cholesterol and volume of aqueous phase. | CCD enhanced amikacin nanoparticles, reducing size, improving loading. |
Development and Characterization of GemcitabineHydrochloride Loaded Lipid Polymer Hybrid Nanoparticles (Lphns) Using Central Composite Design.34 | PLGA 50:50/PLGA 65:35 mass ratio SPC/polymer mass ratio Amount of DSPE-PEG. | CCD optimized ratios for size-reduced LPHN formulation. | |
Solid lipid nanoparticles loaded with nisoldipine, formulated using CCD, underwent testing to evaluate their pharmacokinetic and pharmacodynamic properties.35 | Lipid and egg lecithin. | Oral bioavailability and particle size reduced with CCD. | |
Transdermal Patches. | To enhance the duration and efficacy of carvedilol’s antihypertensive effects, a central composite design should be developed for constructing a transdermal ethosomal hydrogel.36 | Phospholipid, ethanol and propylene glycol. | CCD optimized particle size, drug release and properties. |
Utilizing Design Expert® Software for Developing and Assessing a Controlled Release Transdermal Drug Delivery System of Carvedilol for Hypertension Management.37 | Cholesterol and Lipid, Glycerol. | Improved transdermal distribution and entrapment efficiency with CCD-optimized formulation. | |
Oral Disintegrating Tablets: Oro dispersible tablet. | Formulation optimization of orally disintegrating tablets containing solid dispersion of felodipine and hydroxypropyl methylcellulose using face-centered central composite design.38 | Crospovidone XL and Mannitol. | CCD optimized tablet friability, drug release and disintegration. |
To enhance the formulation and physical attributes of on-demand orodispersible films, the quality-by- design approach is utilized.39 | Drying temperature and Percentage of HPMC and glycerol. | Researchers optimized orodispersible films by evaluating various factors. | |
Microspheres | Quality by Design (QbD) study on enoxaparin sodium-loaded polymeric microspheres for colon-specific delivery.40 | The PVA content in the external phase, the combination ratio of Eudragit® FS-30D to Eudragit® RS-PO and the concentration of NaCl in the external phase. | CCD optimized microsphere size, enhanced enoxaparin encapsulation and controlled release in various media. |
Analysis of dextromethorphan using UPLC. | Using an innovative combination of quality-by-design and green analytical chemistry approaches for the development of a stability-indicating UHPLC method in pharmaceutical products.41 | pH, gradient slope, flow rate and temperature. | Enhancements improved UHPLC method efficiency and stability assessment. |
Electrophoresis analysis. | New trends in the quality control of enantiomeric drugs: quality by design-compliant development of chiral capillary electrophoresis methods.42 | Cyclodextrin concentration, pH and voltage. | Resolution and analysis time in cyclodextrin-modified micellar electrokinetic chromatography. |
Ophthalmic Drug Delivery Systems. | Formulation and development of ophthalmic in situ gel for treating ocular inflammation and infection using the application of quality by design concept.43 | Poloxamer 407, hydroxyl propyl methyl cellulose (HPMC) K4M. | In situ gel for CCD increased drug exposure in rabbits. |
Gastro retentive Drug Delivery Systems. | Creating Gastro retentive Mucoadhesive Microspheres of Repaglinide: Employing a Design of Experiment Approach for Preparation, Characterization and Performance Enhancement.44 | Methocel K15M Eudragit L 100 RPM. | CCD optimized formulation achieved superior drug release and binding. |
Gastro retentive Drug Delivery Systems. | QbD-enabled development of novel stimuli-responsive gastro-retentive systems of acyclovir for improved patient compliance and biopharmaceutical performance.45 | sodium alginate and gelatin. | CCD presence enhanced viscosity, gel strength, flotation and drug release. |
Hydrogels for Topical Delivery. | Formulation and optimization of gentamicin hydrogel infused with Tetracarpidium conophorum extract via a central composite design for topical delivery.46 | Transcutol, stirring speed, flux. | Optimizing gentamicin hydrogel with CCD for drug penetration. |
Experimental Design and Data Analysis
Steps Involved in Designing Experiments Using CCD
CCD is a systematic approach for designing experiments, to understand and to optimize the relationship between independent variables and a system’s response. The process involves several key steps, such as identifying factors and their levels, selecting center and axial points, determining alpha values, creating experimental runs, randomizing the order, conducting experiments, analyzing data using regression modeling, and checking the adequacy of the model.47 This approach facilitates the efficient exploration of the response surface, identifying optimal conditions and supporting informed decision-making in various processes (Figure 5).
Statistical Methods for Data Analysis, Including Regression Analysis and Model Fitting
Statistical methods are crucial in extracting valuable insights from CCD experiments, aiding researchers in comprehending the connections between factors and responses, refining formulations and making informed decisions in pharmaceutical science and related fields. The subsequent section outlines the statistical methods utilized in CCD.
Top of Form
Analyzing Regression
CCD creates models that can predict outcomes based on various factors. Linear regression helps estimate coefficients for different factors, showing how each affects the outcome. On the other hand, quadratic regression includes quadratic terms to account for non-linear relationships, providing a more precise understanding of complex interactions.48 This approach is particularly important in pharmaceutical science and other research areas where relationships between variables are intricate.
Model Fitting
Response Surface Methodology (RSM) relies on CCD to model response surfaces accurately based on experimental data.49 The primary aim of RSM is to develop mathematical models that depict the relationship between various factors and their corresponding responses. These models are instrumental in determining the most favorable conditions for a given process or system.
ANOVA
ANOVA is employed to evaluate the importance of each factor and interaction term within the regression model, determining whether the model adequately captures the variation in the response variable.50
Model Adequacy Checking
Residual analysis evaluates model fit by comparing observed and predicted values, aiming for normal distribution and pattern-free residuals. These tests help ascertain whether the model accurately represents the true relationship between factors and responses.51
Optimization Techniques
The desirability functional approach offers a way to optimize multiple responses concurrently. This method aids in pinpointing factor settings that yield the most favorable combination of response variables. Constraint optimization refers to constraints on factor levels that determine the best factor settings while adhering to these constraints.52
Variable Selection
Stepwise Regression identifies crucial factors for inclusion, streamlining models without compromising accuracy. Well-structured experimental designs improve precision by reducing bias and managing variables, enhancing internal validity. Ensuring sample representativeness and controlling variables increase external validity, making results widely applicable. Furthermore, a carefully planned experiment helps optimize the use of resources, such as time and materials, leading to greater efficiency in research efforts.53
Benefits and Advantages of CCD
Benefits of Using CCD in Pharmaceutical Research
CCD optimizes pharmaceutical formulations by systematically varying factors like excipients and processing conditions, enhancing product quality and understanding complex behaviors.54 CCD aligns with QbD principles as it helps researcher’s pinpoint critical formulation and process parameters,55 promoting a systematic pharmaceutical development approach focusing on understanding product and process variability. Overall, CCD enables pharmaceutical scientists to navigate the complexities of drug development, offering a systematic approach for making well-informed decisions, whether in nanoparticle formulation, protein stability studies, or process optimization.56
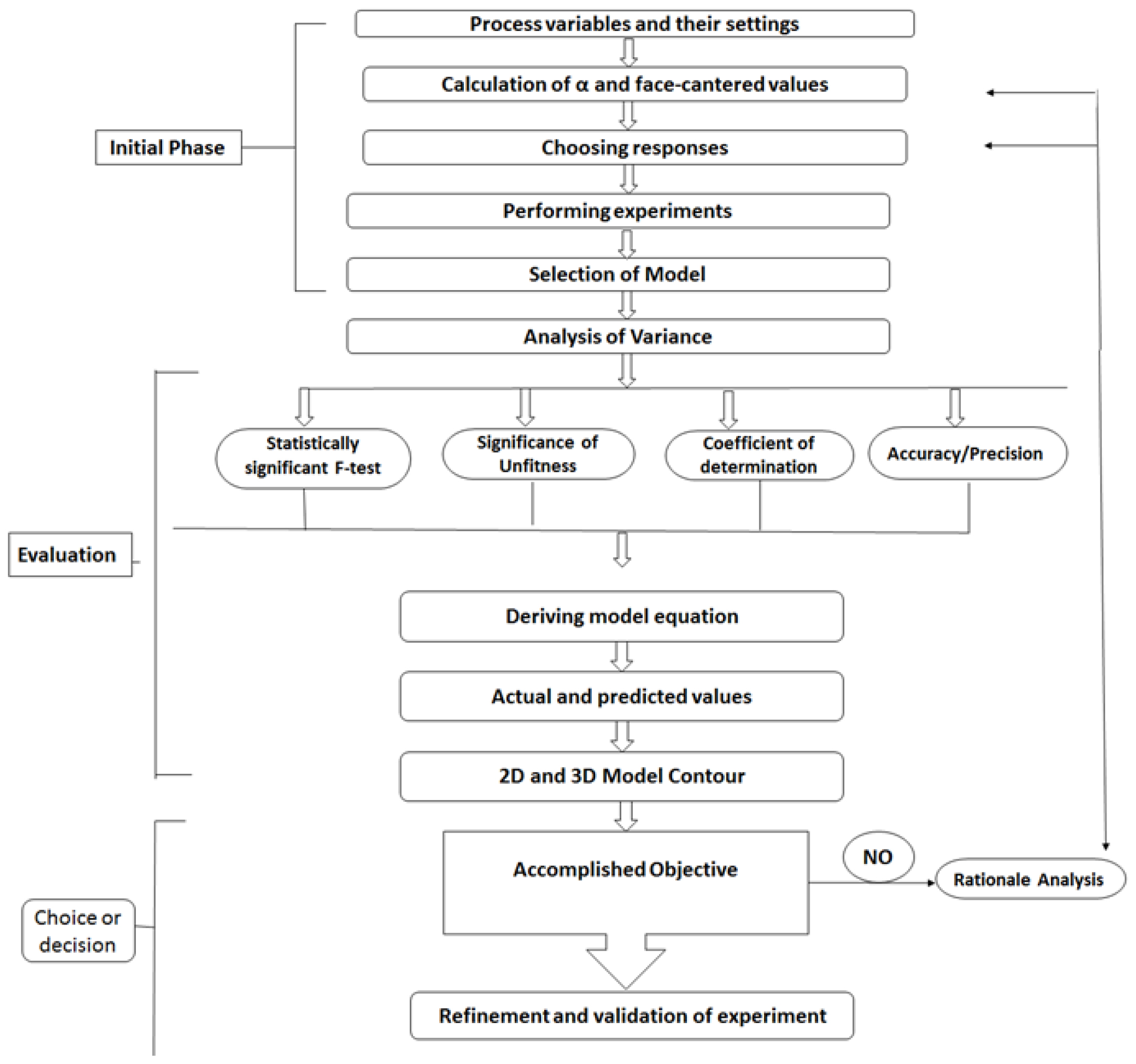
Figure 5:
Flow chart for steps in CCD.
CCD Streamlines the Optimization Process, leading to Cost and Time Savings
CCD extends 2-level factorial or fractional factorial designs to evaluate nonlinearity in a dataset. It helps estimate curvature in continuous responses, enabling the exploration of complex relationships in experimental data. This approach is advantageous because it extracts maximum information from minimal experimental trials. In essence, CCD is a versatile tool for researchers aiming to analyze nonlinear and continuous responses in their experimental investigations efficiently and comprehensively.57
CCD In Robust And Reproducible Formulations
Central Composite Design optimizes formulation outcomes by systematically exploring factor impacts, enhancing dependability and reproducibility. Despite challenges, CCD is valuable for stable pharmaceutical formulations, offering a systematic framework for optimization.
Integration with Quality by Design (QbD)
QbD represents a comprehensive methodology for pharmaceutical development. CCD is frequently utilized in QbD and plays a crucial role in optimizing and comprehending the influence of different factors on the ultimate quality of the product.57 CCD proves valuable in analyzing the relationship between several independent variables and the system’s response (output).58 The approach integrates QbD with Central Composite Design for studying variable effects, optimal conditions and interactions in pharmaceutical and manufacturing processes. It uses statistical tools such as Response Surface Methodology (RSM) to model and optimize the relationship between variables and product quality.59 CCD ensures robust production, linking closely with QbD for optimization. CCD is a valuable tool within the QbD paradigm, aiding in experimental design, data collection and statistical analysis to understand and optimize the factors influencing product quality.60
Regulatory Considerations
Regulatory bodies like Faster Data Analysis require thorough evidence of drug development processes to ensure safety, efficacy and reproducibility.61 DOE and ICH Q8 (R2) promote systematic, science-based pharmaceutical development. ICH Q9 provides principles for implementing a systematic and science-based quality risk management process in the pharmaceutical industry.62 The USP General Chapter on Biological Assay Validation provides guidelines for incorporating DOE in the validation process, emphasizing its relevance to biopharmaceuticals. ASTM International standards focus on statistical methods, specifically for DOE and adhere to regulatory principles, documentation and data integrity.63
CONCLUSION
Central Composite Design is vital in pharmaceutical research, aiding in drug formulation and process optimization, aligning with Quality by Design principles. It integrates with multivariate analysis and innovative technologies like AI. Challenges include complex data handling, limited exploration of extreme conditions, symmetry assumptions and sensitivity to outliers. Despite challenges, CCD remains potent, aiding efficient decision-making in drug development. To enhance its efficacy, researchers should improve statistical abilities, foster interdisciplinary collaboration, leverage emerging technologies like AI, conduct thorough risk assessments and carefully consider extreme conditions. These strategies can enhance CCD experiments and optimize drug formulations.
Cite this article:
Sruthi S, Gopinath S, Stanley MA, Kumar SS. Central Composite Design: An Optimization Tool for Developing Pharmaceutical Formulations. J Young Pharm. 2024;16(3):400-9.
ACKNOWLEDGEMENT
The authors are thankful to Sri Ramachandra Institute of Higher Education and Research (DU), Porur, Chennai.
References
- Ahmadi M, Vahabzadeh F, Bonakdarpour B, Mofarrah E, Mehranian M. Application of the central composite design and response surface methodology to the advanced treatment of olive oil processing wastewater using Fenton’s peroxidation. J Hazard Mater. 2005;123(1-3):187-95. [PubMed] | [CrossRef] | [Google Scholar]
- Sarrai AE, Hanini S, Merzouk NK, Tassalit D, Szabó T, Hernádi K, et al. Using central composite experimental design to optimize the degradation of Tylosin from aqueous solution by photo-fenton reaction. Materials (Basel). 2016;9(6):428 [PubMed] | [CrossRef] | [Google Scholar]
- Gujral G, Kapoor D, Jaimini M. An updated review on design of experiment (DOE) in pharmaceuticals. J Drug Deliv Ther. 2018;8(3):147-52. [CrossRef] | [Google Scholar]
- Ahn H. In: Ind eng management sci appl. 2015;2015:969-79. [CrossRef] | [Google Scholar]
- Savic Gajic I, Savic I, Boskov I, Žerajíc S, Markovic I, Gajic D, et al. Optimization of ultrasound-assisted extraction of phenolic compounds from black locust () flowers and comparison with conventional methods. Antioxidants (Basel). 2019;8(8):248 [PubMed] | [CrossRef] | [Google Scholar]
- Beg S, Swain S, Rahman M, Hasnain MS, Imam SS. Application of design of experiments (DoE) in pharmaceutical product and process optimization. In: Pharm qual by Des. 2019:43-64. [PubMed] | [CrossRef] | [Google Scholar]
- Sarlak N, Nejad MA, Shakhesi S, Shabani K. Effects of electrospinning parameters on titanium dioxide nanofibers diameter and morphology: an investigation by Box-Wilson Central Composite Design (CCD). Chem Eng J. 2012;210:410-6. [CrossRef] | [Google Scholar]
- Pálfi N, Geier N. A comparative, experimental study of full factorial and central composite designs, through machinability analysis of aluminum alloy. 2019;9(1):11-7. [CrossRef] | [Google Scholar]
- Li Z, Lu D, Gao X. Optimization of mixture proportions by statistical experimental design using response surface method-A review. J Build Eng. 2021;36:102101 [CrossRef] | [Google Scholar]
- Grangeia HB, Silva C, Simões SP, Reis MS. Quality by design in pharmaceutical manufacturing: A systematic review of current status, challenges and future perspectives. Eur J Pharm Biopharm. 2020;147:19-37. [PubMed] | [CrossRef] | [Google Scholar]
- Cox L, Calderón M, Pfaar O. Subcutaneous allergen immunotherapy for allergic disease: examining efficacy, safety and cost-effectiveness of current and novel formulations. Immunotherapy. 2012;4(6):601-16. [PubMed] | [CrossRef] | [Google Scholar]
- Omorogie MO, Naidoo EB, Ofomaja AE. Response surface methodology, Central composite design, process methodology and characterization of pyrolyzed KOH pretreated environmental biomass: mathematical modeling and optimization approach. Model Earth Syst Environ. 2017;3(3):1171-86. [CrossRef] | [Google Scholar]
- Szpisják-Gulyás N, Al-Tayawi AN, Horváth ZH, László Z, Kertész S, Hodúr C, et al. Methods for experimental design, central composite design and the Box-Behnken design, to optimize operational parameters: a review. Acta Aliment. 2023;52(4):521-37. [CrossRef] | [Google Scholar]
- Elsayed EW, Emam MF. Application of response surface methodology using face-centered central composite design for studying long-term stability of gliclazide-loaded multiparticulate systems. J Pharm Sci. 2024 [PubMed] | [CrossRef] | [Google Scholar]
- Monton C, Luprasong C. Effect of temperature and duration time of maceration on nitrate content of (L.) Less.: circumscribed central composite design and method validation. Int J Food Sci. 2019;2019:1281635 [PubMed] | [CrossRef] | [Google Scholar]
- Nwanya JC, Dozie KC. Optimal prediction variance capabilities of inscribed central composite designs. Asian J Probab Stat. 2020;8(1):1-8. [CrossRef] | [Google Scholar]
- Zhang Y, Zhang J. Optimization of headspace solid-phase microextraction for analysis of ethyl carbamate in alcoholic beverages using a face-centered cube central composite design. Anal Chim Acta. 2008;627(2):212-8. [PubMed] | [CrossRef] | [Google Scholar]
- Tarapara B, Divecha J. Practical efficient alpha: encompassing all alphas of central composite design. Commun Stat Simul Comput. 2023;52(4):1621-9. [CrossRef] | [Google Scholar]
- Pramod K, Tahir MA, Charoo NA, Ansari SH, Ali J. Pharmaceutical product development: A quality by design approach. Int J Pharm Investig. 2016;6(3):129-38. [PubMed] | [CrossRef] | [Google Scholar]
- Mishra V, Thakur S, Patil A, Shukla A. Quality by design (QbD) approaches in current pharmaceutical set-up. Expert Opin Drug Deliv. 2018;15(8):737-58. [PubMed] | [CrossRef] | [Google Scholar]
- Meka VS, Thing LK, Gorajana A, Kolapalli VR. Formulation and optimization of gastric floating drug delivery system using Central composite design and its biopharmaceutical evaluation. Pak J Pharm Sci. 2015;28(4):1373-87. [PubMed] | [Google Scholar]
- Liu Y, Yang G, Jin S, Xu L, Zhao CX. Development of high-drug-loading nanoparticles. ChemPlusChem. 2020;85(9):2143-57. [PubMed] | [CrossRef] | [Google Scholar]
- Kumar P, Singh C. A study on solubility enhancement methods for poorly water-soluble drugs. Am J Pharmacol Sci. 2013;1(4):67-73. [CrossRef] | [Google Scholar]
- Teżyk M, Jakubowska E, Milanowski B, Lulek J. Implementation of quality by design approach in manufacturing process optimization of dry granulated, immediate release, coated tablets-a case study. Drug Dev Ind Pharm. 2017;43(10):1626-36. [PubMed] | [CrossRef] | [Google Scholar]
- Vuppalapati L, Cherukuri S, Neeli V, Reddy Yeragamreddy PY, Reddy Kesavan B. Application of central composite design in optimization of valsartan nanosuspension to enhance its solubility and stability. Curr Drug Deliv. 2016;13(1):143-57. [CrossRef] | [Google Scholar]
- El-Say KM. Maximizing the encapsulation efficiency and the bioavailability of controlled-release cetirizine microspheres using Draper-Lin small composite design. Drug Des Dev Ther. 2016;10:825-39. [CrossRef] | [Google Scholar]
- Aktas E, Eroglu H, Kockan U, Oner L. Systematic development of pH-independent controlled release tablets of carvedilol using a central composite design and artificial neural networks. Drug Dev Ind Pharm. 2013;39(8):1207-16. [PubMed] | [CrossRef] | [Google Scholar]
- Dudhipala N, Veerabrahma K. Pharmacokinetic and pharmacodynamic studies of nisoldipine-loaded solid lipid nanoparticles developed by central composite design. Drug Dev Ind Pharm. 2015;41(12):1968-77. [PubMed] | [CrossRef] | [Google Scholar]
- Montazer M, Taghavi FA, Toliyat T, Moghadam MB. Optimization of dyeing of wool with madder and liposomes by central composite design. J Appl Polym Sci. 2007;106(3):1614-21. [CrossRef] | [Google Scholar]
- Karemore MN, Avari JG. Formulation, optimization and evaluation of gastroretentive drug delivery system of nifedipine for treating preeclampsia. AAPS PharmSciTech. 2019;20:1-6. [CrossRef] | [Google Scholar]
- Singh B, Chakkal SK, Ahuja N. Formulation and optimization of controlled release mucoadhesive atenolol tablets using response surface methodology. AAPS PharmSciTech. 2006;7(1):E19-28. [CrossRef] | [Google Scholar]
- Zeng C, Jiang W, Tan M, Yang X, He C, Huang W, et al. Optimization of the process variables of tilianinloaded composite phospholipid liposomes based on response surface-central composite design and pharmacokinetic study. Eur J Pharm Sci. 2016;88:80-9. [CrossRef] | [Google Scholar]
- Varshosaz J, Ghaffari S, Khoshayand MR, Atyabi F, Azarmi S, Kobarfard F, et al. Development and optimization of solid lipid nanoparticles of amikacin by central composite design. J Liposome Res. 2010;20(2):97-104. [PubMed] | [CrossRef] | [Google Scholar]
- Yalcin TE, Ilbasmis-Tamer S, Takka S. Development and characterization of gemcitabine hydrochloride loaded lipid polymer hybrid nanoparticles (dolphins) using central composite design. Int J Pharm. 2018;548(1):255-62. [PubMed] | [CrossRef] | [Google Scholar]
- Dudhipala N, Veerabrahma K. Pharmacokinetic and pharmacodynamic studies of nisoldipine-loaded solid lipid nanoparticles developed by central composite design. Drug Dev Ind Pharm. 2015;41(12):1968-77. [PubMed] | [CrossRef] | [Google Scholar]
- Amarachinta PR, Sharma G, Samed N, Chettupalli AK, Alle M, Kim JC, et al. Central composite design for developing carvedilol-loaded transdermal ethosomal hydrogel for extended and enhanced antihypertensive effect. J Nanobiotechnology. 2021;19:1-5. [PubMed] | [CrossRef] | [Google Scholar]
- Maan S, Khar RK, Mazumder R, Yadav N, Khan UA. Fabrication and evaluation of controlled release transdermal drug delivery system of carvedilol using Design Expert® software for the management of hypertension. J Young Pharm. 2022;14(3):295-301. [CrossRef] | [Google Scholar]
- Tung NT, Hung MV, Vo XM, Nguyen TH, Pham TM. Formulation optimization of orally disintegrating tablets containing solid dispersion of felodipine and hydroxypropyl methylcellulose using face-centered central composite design. J Pharm Investig. 2014;44(2):111-8. [CrossRef] | [Google Scholar]
- Visser JC, Dohmen WM, Hinrichs WL, Breitkreutz J, Frijlink HW, Woerdenbag HJ, et al. Quality by design approach for optimizing extemporaneously prepared orodispersible films’ formulation and physical properties. Int J Pharm. 2015;485(1-2):70-6. [PubMed] | [CrossRef] | [Google Scholar]
- Hales D, Vlase L, Porav SA, Bodoki A, Barbu-Tudoran L, Achim M, et al. Quality by design (QbD) study on enoxaparin sodium-loaded polymeric microspheres for colon-specific delivery. Eur J Pharm Sci. 2017;100:249-61. [PubMed] | [CrossRef] | [Google Scholar]
- Boussès C, Ferey L, Vedrines E, Gaudin K. Using an innovative combination of quality-by-design and green analytical chemistry approaches to develop a stability-indicating UHPLC method in pharmaceutical products. J Pharm Biomed Anal. 2015;115:114-22. [CrossRef] | [Google Scholar]
- Orlandini S, Hancu G, Szabó ZI, Modroiu A, Papp LA, Gotti R, et al. New trends in the quality control of enantiomeric drugs: quality by design-compliant development of chiral capillary electrophoresis methods. Molecules. 2022;27(20):7058 [PubMed] | [CrossRef] | [Google Scholar]
- Patel N, Thakkar V, Metalia V, Baldaniya L, Gandhi T, Gohel M, et al. Formulation and development of ophthalmic gel for the treatment ocular inflammation and infection using application of quality by design concept. Drug Dev Ind Pharm. 2016;42(9):1406-23. [PubMed] | [CrossRef] | [Google Scholar]
- Bhowmick T, Saha JR, Karmoker MS. Reza, Design of the experiment (doe) approach to prepare, characterize and optimize the gastro retentive mucoadhesive microspheres of repaglinide. Bangladesh Pharm J. 2019;22(2):135-45. [PubMed] | [CrossRef] | [Google Scholar]
- Singh A, Kaur S, Dhiman B, Garg RKK, Beg S. QbD-enabled development of novel stimuli-responsive gastro retentive systems of acyclovir for improved patient compliance and biopharmaceutical performance. AAPS PharmSciTech. 2016;17:454-65. [CrossRef] | [Google Scholar]
- Ilomuanya MO, Amenaghawon NA, Odimegwu J, Okubanjo OO, Aghaizu C, Oluwatobiloba A, et al. Formulation and optimization of gentamicin hydrogel infused with Tetracarpidium conophorum extract via a central composite design for topical delivery. Turk J Pharm Sci. 2018;15(3):319-27. [PubMed] | [CrossRef] | [Google Scholar]
- Heinisch J, Lockner Y, Hopmann C. Comparison of design of experiment methods for modeling injection molding experiments using artificial neural networks. J Manuf Processes. 2021;61:357-68. [CrossRef] | [Google Scholar]
- Nooraziah VJ. Tiagrajah, A study on a regression model using response surface methodology. Appl Mech Mater. 2014;666:235-9. [CrossRef] | [Google Scholar]
- Shalabh C, Heumann SH, Park HJ, Kim JI. Cho, Optimal central composite designs for fitting second-order response surface linear regression models. In: Recent advances in linear models and related areas: essays in honour of Helge Toutenburg. 2008:323-39. [CrossRef] | [Google Scholar]
- Chen WH, Carrera Uribe MC, Kwon EE, Lin KY, Park YK, Ding L, et al. A comprehensive review of thermoelectric generation optimization by statistical approach: Taguchi method, analysis of variance (ANOVA) and response surface methodology (RSM). Renew Sustain Energy Rev. 2022;169:112917 [CrossRef] | [Google Scholar]
- Harrell FE, Lee KL, Mark DB. Multivariable prognostic models: issues in developing models, evaluating assumptions and adequacy and measuring and reducing errors. Stat Med. 1996;15(4):361-87. [PubMed] | [CrossRef] | [Google Scholar]
- Rukmana AY, Meltareza R, Harto B, Komalasari O, Harnani N. Optimizing the role of business incubators in higher education: a review of supporting factors and barriers, west sci. Bus Manag. 2023;1(03):169-75. [PubMed] | [CrossRef] | [Google Scholar]
- Chong SS, Aziz AA, Harun SW, Arof H, Shamshirband S. Application of multiple linear regression, central composite design and ANFIS models in dye concentration measurement and prediction using plastic optical fiber sensor. Measurement. 2015;74:78-86. [CrossRef] | [Google Scholar]
- Peng Soh JL, Liew CV, Sia Heng PW. Impact of excipient variability on drug product processing and performance. Curr Pharm Des. 2015;21(40):5890-9. [CrossRef] | [Google Scholar]
- Pramod K, Tahir MA, Charoo NA, Ansari SH, Ali J. Pharmaceutical product development. A quality by design approach. Int J Pharm Investig. 2016;6(3):129-38. [CrossRef] | [Google Scholar]
- Politis SN, Colombo P, Colombo G, Rekkas DM. Design of experiments (DoE) in pharmaceutical development. Drug Dev Ind Pharm. 2017;43(6):889-901. [CrossRef] | [Google Scholar]
- Fukuda IM, Pinto CF, Moreira CS, Saviano AM, Lourenço FR. Design of experiments (DoE) applied to pharmaceutical and analytical quality by design (QbD). Braz J Pharm Sci. 2018;54(spe) [CrossRef] | [Google Scholar]
- Ferreira SL, Bruns RE, da Silva EG, Dos Santos WN, Quintella CM, David JM, et al. Statistical designs and response surface techniques for the optimization of chromatographic systems. J Chromatogr A. 2007;1158(1-2):2-14. [PubMed] | [CrossRef] | [Google Scholar]
- Witek-Krowiak A, Chojnacka K, Podstawczyk D, Dawiec A, Bubała K. Application of response surface methodology and artificial neural network methods in modeling and optimizing biosorption process. Bioresour Technol. 2014;160:150-60. [PubMed] | [CrossRef] | [Google Scholar]
- Herwig C, Garcia-Aponte OF, Golabgir AS, Rathore AS. Knowledge management in the QbD paradigm: manufacturing of biotech therapeutics. Trends Biotechnol. 2015;33(7):381-7. [PubMed] | [CrossRef] | [Google Scholar]
- Sangshetti JN, Deshpande M, Zaheer Z, Shinde DB, Arote R. Quality by design approach: regulatory need. Arab J Chem. 2017;10:S3412-25. [CrossRef] | [Google Scholar]
- Karanakov L, Tonic-Ribarska J, Glavas-Dodov M, Trajkovic-Jolevska S. Analysis and critical review of ICH Q8, Q9 and Q10 from a generic pharmaceutical industry viewpoint. J Geophys Res Solid Earth. 2012;93:10174-90. [CrossRef] | [Google Scholar]
- Alexander AE, Wake N, Chepelev L, Brantner P, Ryan J, Wang KC, et al. A guideline for 3D printing terminology in biomedical research utilizing ISO/ASTM standards. 3D Print Med. 2021;7:1-6. [CrossRef] | [Google Scholar]